In a World (Built by Machines)
by Phil Bernstein
Associate Dean and Professor Adjunct, Yale University
August 10, 2022
A DesignIntelligence conversation with Phil Bernstein about his new book, “Machine Learning: Architecture in the Age of Artificial Intelligence”
DesignIntelligence (DI): We’re here to talk about your new book, “Machine Learning: Architecture in the Age of Artificial Intelligence,” just published by RIBA. First off, I have to say, I love this book. It’s scholarly, well researched, thoughtfully organized, concisely written and beautifully designed into three clear sections plus a conclusion. I very much like the “matrix” table of contents diagram, the use of color throughout — and a stellar set of clarifying diagrams. Truth be told, I read it in one sitting and filled the pages with marginalia. It was a delight. But enough gushing and congratulations.
Phil Bernstein (PB): Thanks, Michael — glad it was both pleasurable and short! As you know, I’m a fan of direct clarity, so I’m happy that’s how you saw the project.
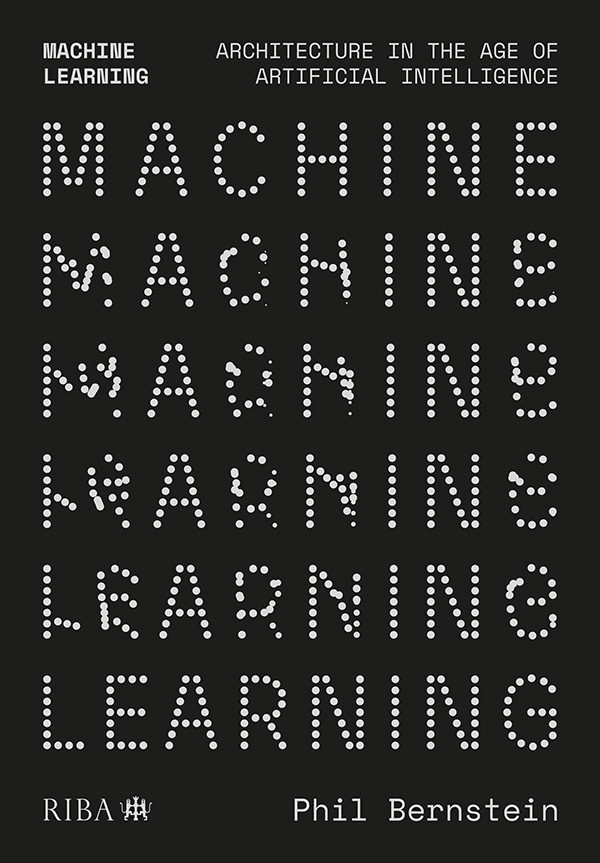
Cover image, courtesy of Phil Bernstein.
DI: I chose this book and a discussion with you for several reasons. First, your book is timely and necessary as architecture seeks to redefine itself in a post-COVID-19 world that is more technology-enabled, more inclusive and more sustainable, and do it in ways that offer more value to customers and more influence by design professionals. The second reason is that your book fits perfectly with our editorial roadmap for this quarter: world building. And third, because not only have you been a leading voice for digital practice and change in the design and construction industry for decades, but you’re always fun to talk to. I know you’ll shoot straight and won’t be timid about being provocative. Let’s talk about this new book. What sparked it?
PB: I was initially contacted by the Royal Institute of British Architects, where I’ve been a longtime member, to see if I might have some thoughts on the topic. The request made me remember I had started working on artificial intelligence (AI) in college, where I studied with one of the founders of natural language processing theory, a then-young professor named Roger Schanck. In my first job out of grad school, I did some brief research on the potential for what were then called “expert systems,” but on the cusp of the first so-called “AI Winter,” there wasn’t much “there” there. It’s only been in the last few years that the technology has matured to the point of being of interest to architects. Today, we have both the hardware (super-fast GPUs, the cloud, the internet) and the software (neural networks) to make some interesting things possible. Back then, when I was coding LISP on a PDP-11, not so much.
DI: I’m guessing most of our readers at DesignIntelligence, many of whom are boomers, won’t know much about AI or machine learning (ML). I know I don’t. For those who haven’t read your book yet, can you give us an overview baseline definition or some applied examples of how it might be used in a design effort?
PB: Let’s start with a distinction I make in the book between computation that automates processes versus those that are autonomous. An algorithm that’s automated might insert a door object into a wall object where you tell it in a building information model (BIM), so you don’t have to take all the steps, even though they are digital, to make that happen. AI-based algorithms are based on logic that allows them to “understand” (within limits) the context in which they are operating and make “decisions” autonomously within that context. When you place that door in your BIM, that’s automation. When the machine decides where the door might best be placed and recommends three options, that’s autonomous computation.
When that program learns your preferences for door locations (or learns them from many other doors it might find in digital form), that’s a subset of artificial intelligence called machine learning. The AI world is further divided into the so-called “symbolists,” who think that insight needs to be encoded explicitly by rules, and the “connectionists” who believe that if you build a system that can learn about the world by absorbing massive information about it, you don’t need rules. The connectionists have led the machine learning efforts overall.
DI: Thank you for exposing me to a new expression, what you’ve termed the “computational interregnum,” a gap between eras of technology dominance for the building industry. It seems we might be experiencing a series of interregna. In practice we are searching for direction amid increasing pressures you cite in the book: a pandemic, social, economic and certainly environmental pressures. Life in every aspect seems to be about uncertainty these days. How is this digital interruption different what we’ve experienced over the last 40 years?
PB: You’re waxing more political and philosophical than my observation, which was about the fact that AEC technology has, until recently, been largely dominated by “hero solutions,” beginning with the early days of AutoCAD through the BIM era. But as the industry has been more broadly digitized, we’re now reliant on a whole range of digital tools, very few of which are related; neither can they work together. That explosion of applications and their data will continue, and the interregnum is the period during which the breadth of digital data far exceeds our ability to make complete sense of it, but it will end with the advent of widespread machine autonomy. My proposal is that AI will not just create autonomous functionality, but also platforms for organizing, integrating, accessing, and reasoning about all that information. It may be easier for an AI to figure out that a door object in a BIM, an entry in a door schedule, that same door on a shop drawing and again on an invoice are related phenomena than trying to build some sort of intergalactic interoperability schema to do so.
As for “uncertainty” writ large, I’ll leave those existential questions to others, but I’ve been around long enough to have seen this condition in the architecture profession since I started my career. Whether it’s risk, span of control, economic uncertainty, climate change or digital replacement, it’s always something. Yet the profession continues to thrive, and there is a steady stream of talented students who wish to join it. So, maybe things aren’t so uncertain after all, and that uneasiness is a more fundamental characteristic of our profession.
DI: You acknowledge that the advent and acceptance of technology in architecture has been reluctant at best. We’ve had 30 or 40 years of gestation in CAD, BIM and now the earliest emergence of AI/ML you write about. Quoting you, “the age-old pathologies persist.” As you assert (in addition to your references to the Susskinds’ work), “it is highly unlikely that AI will supplant the practice of architecture in the near future.”
You suggest a task-based focus rather than a wholesale replacement of architects. I have a few challenges to some of the use cases for automated tasks you list in Chapter 3.3., specifically, market conditions, costs, supply chain conditions, construction scheduling and build strategy. Having lived inside a national construction management firm for 22 years, I know how trade-contractor and company-specific each of these knowledge sets are. Each is different. I’m skeptical they can be generated by data and machines across the industry. They are proprietary and localized. Company A has two years’ backlog. Company B needs work. Their outlooks on these potential AI use cases are radically different. Ditto with costs; it’s always contextual. Automated industry cost data like RS Means is generic (read: useless). If I’m right, it doesn’t seem to leave many potential avenues open, at least in the short-term, for AI uses in AE firms. Convince me.
PB: For your first inquiry about the Susskinds, remember that Daniel (the son of Richard) Susskind wrote a second book, “A World Without Work,” where his thinking evolved from the first book (“The Future of the Professions”). The original thesis — that professionals will be replaced by the capabilities of the internet and AI — was succeeded by the idea that it’s not specific jobs that will disappear, especially at the high end of employment like architects, but rather individual tasks that will be replaced, until eventually, broad swathes of jobs will be gone and universal basic income will become a necessity.
My book argues that we should guide the technology toward the most important tasks that can augment — rather than replace — architects, and there are plenty of interesting opportunities to do that.
Some of those opportunities will eventually reach your friends the subcontractors when and if, during the interregnum, the industry — not individual companies — can figure out a way to compile, curate, anonymize and access a compilation of industry-wide data. That’s what it will take to make that data rich enough to train the AIs and interesting enough to these companies, who don’t have enough data within their own shops anyway. So, I agree the short-term uses are probably augmenting current processes (much the same way your phone guesses what you’re typing), but the longer-term possibilities are more interesting.
In any event, AI technologies are accelerating so fast that it’s hard to know what the “short-term” is, exactly. Those same natural language AIs that currently empower your Alexa or write weird poetry have also been trained to write semi-decent Python code. Suddenly, your subs are programmers! Where might that lead?
DI: That’s fascinating to contemplate subcontractors who can write code. I’m guessing some already are. Based on our 40-year backstory of slow technology growth in architecture, why should practitioners care about AI/ML if it’s another 30 years out, to use a Tom Friedman acronym, “IBG/YBG,” or “I’ll be gone/you’ll be gone”? Obviously, as an evangelist and tech seer, you’re suggesting it as a strategy for the leading adopters — those who will lead the charge in adapting.
PB: There are two issues here. First, like most AI books, mine was moving toward obsolescence by the time I wrote it, especially regarding the combination of natural language and image generation. Unlike the CAD-to-BIM transition, which in hindsight turned out — to my great disappointment — to be just another swap of one digital tool for drawing (CAD) to another (BIM), AI technology can learn to do stuff. It’s not a static tool. Further, today’s clients in many industries — health care, finance, real estate, manufacturing, marketing, even law — are starting to use AI to augment and underpin their processes and will ask architects and engineers to do the same. IBG/YBG might apply for you and me, my fellow Boomer, but not for my students or, frankly, even midcareer professionals.
DI: As longtime technology supporters, you and I understand all too well the litany of limitations on broader, faster technology adaptation in architecture. Low profitability (versus other industries), cultural taboos, “we don’t like numbers,” a bias against “numeracy” as Z. Smith at EskewDumas Ripple called it. We architects generally don’t like and aren’t good at quantifying things or proving performance metrics. I loved your benchmarking us to law and medicine, both of which have long-standing central shared databases. But architecture? Nada. You outline what it will take to get us over these humps in the book’s conclusion. Why is this so important? What will it take to get us there?
PB: There is a difference between “not liking to quantify things” and “not converting the value of doing so” in current delivery and business models. Maybe a certain generation of architects “don’t like to do it,” but that’s fading with the current, numerate, digitally-enabled generation, and the social operating context — which is increasingly dependent on digital analysis and validation — that’s changing, as I mentioned above. In the book, I describe a strategy for a national AEC data trust curated by a third party, which would allow us to compile and access our data collectively, much like Westlaw (for the lawyers) or the FDA’s DailyMed drug interaction database (for doctors). Further, the demands of current, relevant design that responds to the big issues of climate change, embodied carbon or even social justice (how a building affects the community it inhabits) are analytical problems that can’t be solved with pencil sketches. As the market has taught us repeatedly, if architects won’t do something that really needs to be done, someone else will certainly step up.
DI: Let’s get practical and tactical. I’ll put you on the spot. What should or could a midsize firm do? They’ve read your book. They’re inspired. They’ve got a few young enough folks with the right amount of juice, passion, political capital and time left in their careers to begin such a strategic differentiating change. And let’s assume they are profitable enough to have some R&D money.
What could they do to have some kind of transformation in place by your target year of 2030? Only eight years off now, 10 from when you were writing the book. And I picked the midsize firm because it’s always toughest for them. The big firms have resources, people, funding, history and inertia. The boutique firms can pivot, get venture capital and stay agile and nimble. They’ve shown themselves to be tech leaders. But what do the folks in the middle do if they’re moved to act by your book? The skeptics, the majority, even the laggards … or are they simply doomed?
PB: If I were running a midsize firm, I’d do three things: First, make sure one of my younger, digitally savvy architects is keeping up with what’s happening in the field. Things are moving fast. New capabilities emerge every day and the early birds must be opportunistic about what might work with their current design culture. Second, there are plenty of “user accessible” AI platforms out there to play with, from prototype projects like DALL-E Mini to mainstream infrastructure like GoogleAI or Microsoft Azure. In between are the accessible tools like CreatML (from Apple) or RunwayML (that does image capture and other visual things). I would make sure someone in my shop was playing with these tools and looking for chances to deploy them. Finally, I would listen carefully to what my clients are doing these days to run their own enterprises. AI systems are infiltrating a lot of business and other processes, and those same clients may soon be asking what we’re doing on that same front.
DI: What have I not asked that you’d like to say? The book is done, it’s out. What’s your message to generate momentum?
PB: The last chapter offers the message: The building professions need to start thinking about, and generating together, a strategic direction for AI/ML technologies or we will lose our agency in their development — and, by implication, our own destiny. I know from my time as a vendor that they much prefer coherent, multi-customer declarations of requirements and use cases to their own attempts to define same; it is both more efficient and less risk for them to do so. This approach makes it more likely that architects, engineers and builders will get what they need, rather than what is most opportunistically profitable, in their toolsets.
DI: Phil, thank you for another fun, provocative conversation. You’ve earned your spot as architecture’s technology prophet for good reason. I hope you, and the book, continue to do well. And I hope plenty of energetic architects read it and do something to change our collective lot in the design and construction supply network — by using machines to help us get smarter, better and more valuable as we do what we love.
PB: Thanks, Michael. Always a pleasure to compare notes with you on these sorts of issues!
Phil Bernstein is an architect, technologist and educator who has taught at the Yale School of Architecture since 1988 and where he received his B.A. (honors) and M.Arch. He is currently associate dean and professor adjunct. He was formerly a vice president at Autodesk, responsible for setting the company’s future vision and strategy for BIM technology, as well as cultivating and sustaining the firm’s relationships with strategic industry leaders and associations. Prior to Autodesk, Phil was a principal at Pelli Clarke and Partners, managing many of the firm’s most complex commissions. He is the author of “Machine Learning: Architecture in the Age of Artificial Intelligence” (2022), “Architecture | Design | Data: Practice Competency in the Era of Computation” (2018) and co-editor of “Building (In) The Future: Recasting Labor in Architecture” (2010, with Peggy Deamer), and consults, speaks and writes extensively on technology, practice and project delivery. He is a senior fellow of the Design Futures Council and former chair of the AIA National Contract Documents Committee.